芳香族化合物与水合电子水相反应速率常数的QSAR模型研究
QSAR models for predicting the aqueous reaction rate constants of aromatic compounds with hydrated electrons
-
摘要: 基于水合电子(eaq-)的高级还原技术(ARPs)是一种有效地去除水中痕量有机污染物的技术.有机物与水合电子反应的速率常数(keaq-)是评价高级还原处理体系中有机物去除效率的一个重要参数.然而化学品种类繁多,通过实验方法一一获取keaq-是不现实的.因此,需要建立一种能够快速预测keaq-的方法,填补现有数据的缺失.本研究搜集整理了94种芳香族化合物的keaq-实验值,采用逐步多元线性回归(MLR)和支持向量机(SVM)方法分别构建了预测化合物的keaq-线性和非线性定量结构活性关系(QSAR)模型.两个模型均具有良好的拟合度(Radj,tr2> 0.800)、稳健性(QLOO2=0.782)和预测能力(Qext2> 0.790).机理分析表明,最低未占据分子轨道能(ELUMO)和偶极矩加权的来自扩增边缘邻接处的最大特征值(SpMAD_AEA(dm))是影响有机物同eaq-反应活性的重要参数,此外,keaq-还与化合物的键级和极化率有关.元分析结果表明具有吸电子官能团的芳香化合物比含供电子基团的化合物倾向于与eaq-有更高的反应活性.
-
关键词:
- 芳香族化合物 /
- 水合电子(eaq-) /
- 反应速率常数 /
- 定量结构活性关系(QSAR)
Abstract: Hydrated electron (eaq-)-based reduction processes are promising for degrading organic pollutants in water engineering systems. The second order rate constant (keaq-) of eaq- with organic compounds is an important parameter for evaluating the removal efficiency of organic pollutants in advanced reduction proless. However, experimental determination of keaq-seems fairly unrealistic because of the large number of organic chemicals. Thus, it is necessary to develop an effective method to predict keaq-. In this study, the experimental keaq-values of 94 aromatic compounds were collected. The quantitative structure-activity relationship (QSAR) models for predicting keaq-were constructed by stepwise multiple linear regression (MLR) and support vector machines (SVM) methods, respectively. Both two models had satisfactory goodness-of-fit (Radj,tr2 > 0.800), robustness (QLOO2=0.782) and good predictability (Qext2> 0.790). Mechanistic analysis reveald that the energy of the lowest unoccupied molecular orbital (ELUMO) and the spectral mean absolute deviation from augmented edge adjacency mat weighted by dipole moment (SpMAD_AEA(dm)) were the most important descriptors. Additionally, keaq- was found to be related to the bond order and polarizability of the compounds. The meta-analysis showed that aromatic compounds with electron-withdrawing functional groups tended to have higher reactivity with eaq- than those containing electron-donating groups. -
-
[1] VELLANKI B P, BATCHELOR B, ABDEL-WAHAB A. Advanced reduction processes:A new class of treatment processes[J]. Environmental Engineering Science, 2013, 30(5):264-271. [2] JUNG B, NICOLA R, BATCHELOR B, et al. Effect of low-and medium-pressure Hg UV irradiation on bromate removal in advanced reduction process[J]. Chemosphere, 2014, 117(1):663-672. [3] YAN Q, ZHANG C J, FEL L, et al. Photo-reductive defluorination of perfluorooctanoic acid in water[J]. Water Research, 2010, 44(9):2939-2947. [4] LI X, MA J, LIU G, et al. Efficient reductive dechlorination of monochloroacetic acid by sulfite/UV process[J]. Environmental Science & Technology, 2012, 46(13):7342-7349. [5] YU H, NIE E, XU J, et al. Degradation of diclofenac by advanced oxidation and reduction processes:Kinetic studies, degradation pathways and toxicity assessments[J]. Water Research, 2013, 47(5):1909-1918. [6] ROSSKY P J, SCHNITKER J. The hydrated electron:Quantum simulation of structure, spectroscopy, and dynamics[J]. The Journal of Physical Chemistry, 1988, 92(15):4277-4285. [7] THOMAS-SMITH T E, BLOUGH N V. Photoproduction of hydrated electron from constituents of natural waters[J]. Environmental Science & Technology, 2001, 35(13):2721-2726. [8] MEZYK S P, HELGESON T, COLE S K, et al. Kinetics of hydrated electron and hydroxyl radical reactions with halonitromethanes in water[J]. The Journal of Physical Chemistry, 2006, 110(6):2176-2180. [9] JUDSON R, RICHARD A, DIX D J, et al. The toxicity data landscape for environmental chemicals[J]. Environmental Health Perspectives, 2009, 117(5):685-695. [10] ZHUANG S L, WANG H F, DING K K, et al. Interactions of benzotriazole UV stabilizers with human serum albumin:Atomic insights revealed by biosensors, spectroscopies and molecular dynamics simulations[J]. Chemosphere, 2016, 144:1050-1059. [11] QU R J, LIU H X, FENG M B, et al. Investigation on intra molecular hydrogen bond and some thermodynamic properties of polyhydroxylated anthraquinones[J]. Journal of Chemical & Engineering Data. 2012, 57(9):2442-2455. [12] OECD,Guidance doucument on the validation of (quantitative) structure-activity relationships models[R]. OECD, 2007. [13] LUO X, YANG X, QIAO X, et al. Development of a QSAR model for predicting aqueous reaction rate constants of organic chemicals with hydroxyl radicals[J]. Environmental Science Processes & Impacts, 2017, 19(3):350-356. [14] 徐童,陈景文,李超,等. 气相有机化学品与羟基自由基反应速率常数的QSAR模型[J]. 环境化学,2017, 36(4):703-709. XU T, CHEN J W, LI C, et al. QSAR models for predicting hydroxyl radical reaction rate constants with organic chemicals in the atmosphere[J]. Environmental Chemistry, 2017, 36(4):703-709(in Chinese).
[15] LEE Y, VON GUNTEN U. Quantitative structure-activity relationships (QSARs) for the transformation of organic micropollutants during oxidative water treatment[J]. Water Research, 2012, 46(19):6177-6195. [16] POUTSMA M L. Evolution of structure-reactivity correlations for the hydrogen abstraction reaction by hydroxyl radical and comparison with that by chlorine atom[J]. The Journal of Physical Chemistry A, 2013, 117(30):6433-6449. [17] XIAO R, YE T, WEI Z, et al. Quantitative structure-activity relationship(QSAR)for the oxidation of trace organic contaminants by sulfate radical[J]. Environmental Science & Technology, 2015, 49(22):13394-13402. [18] LI X, ZHAO W, LI J, et al. Development of a model for predicting reaction rate constants of organic chemicals with ozone at different temperatures[J]. Chemosphere, 2013, 92(8):1029-1034. [19] CORTES C, VAPNIK V. Support-vector networks[J]. Machine Learning, 1995, 20(3):273-297. [20] LIU H X, XUE C X, ZHANG R S, et al. Quantitative prediction of logk of peptides in high-performance liquid chromatography based on molecular descriptors by using the heuristic method and support vector machine[J]. Journal of Chemical Information & Computer Scienences, 2004, 44(6):1979-1986. [21] YAO X J, PANAYE A, DOUCET J P, et al. Comparative study of QSAR/QSPR correlations using support vector machines, radial basis function neural networks, and multiple linear regression[J]. Journal of Chemical Information & Computer Sciences, 2004, 44(4):1257-1266. [22] REN Y, LIU H, YAO X, et al. Prediction of ozone tropospheric degradation rate constants by projection pursuit regression[J]. Analytica Chimica Acta, 2007, 589(1):150-158. [23] LUO S, WEI Z, DIONYSIOU D D, et al. Mechanistic insight into reactivity of sulfate radical with aromatic contaminants through single-electron transfer pathway[J]. Chemical Engineering Journal, 2017, 327:1056-1065. [24] KARELSON M, LOBANOV V S, KATRIZKY A R. Quantum-chemical descriptors in QSAR/QSPR studies[J]. Chemical Reviews. 1996, 96(3), 1027-1043 [25] WANG Y, CHEN J, LI X, et al. Estimation of aqueous-phase reaction rate constants of hydroxyl radical with phenols, alkanes and alcohols[J]. Molecular Informatics, 2009, 28(11-12):1309-1316 [26] TODESCHINI R. DRAGON-Software for Molecular Descriptor Calculations (Version 5.4 for Windows). Milan, Italy:Talete srl, 2006. [27] 桑兰芬,杨滢,杜秀华,等. 苯、萘、蒽芳烃分子稳定性和分子极化率规律的理论研究[J]. 化学研究与应用,1996, 8(1):70-72. SANG L F, YANG Y, DU X H. Theoretical study of regularity of stability and polarizability of benzene, naphthalene and anthracene[J]. Chemical Research and Application, 1996, 8(1):70-72(in Chinese).
-
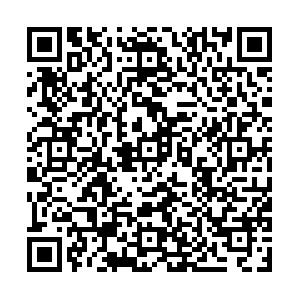
计量
- 文章访问数: 2094
- HTML全文浏览数: 2077
- PDF下载数: 84
- 施引文献: 0